Deep learning method developed to understand how chronic pain affects each patient's body
7/31/23
A research team from the Universidad Carlos III de Madrid (UC3M), together with University College London in the United Kingdom, has carried out a study to analyse how chronic pain affects each patient’s body. Within this framework, a deep learning method has been developed to analyse the biometric data of people with chronic conditions.
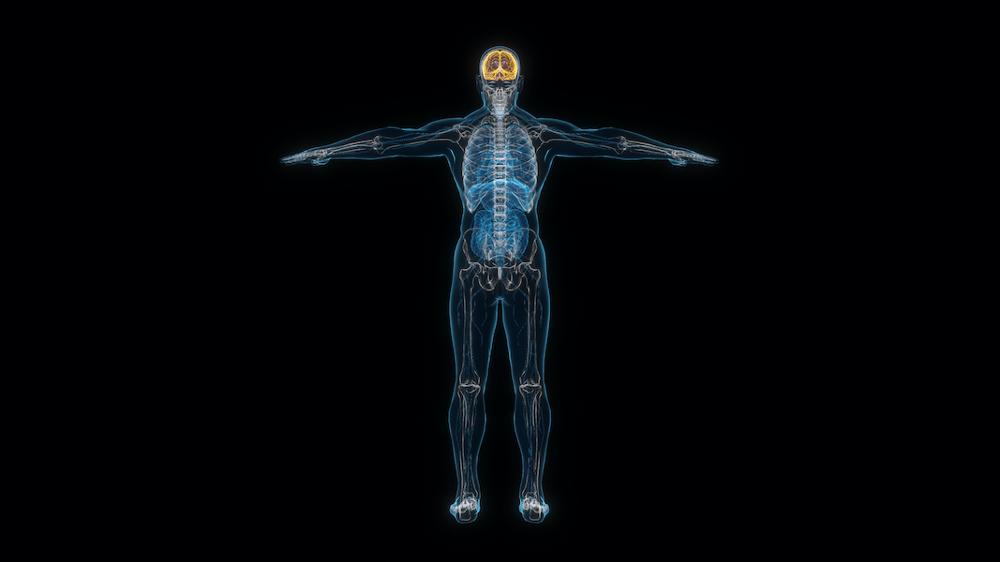
The analysis is based on the hypothesis that people with chronic lower back pain have variations in their biometric data compared to healthy people. These variations are related to body movements or walking patterns and are believed to be due to an adaptive response to avoid further pain or injury.
However, research to date has found it difficult to accurately distinguish these biometric differences between people with and without pain. There have been several factors, such as the scarcity of data related to this issue, the particularities of each type of chronic pain and the inherent complexity in the measurement of biometric variables.
“People with chronic pain often adapt their movements to protect themselves from further pain or injury. This adaptation makes it difficult for conventional biometric analysis methods to accurately capture physiological changes. Hence the need to develop this system”, says Doctor Mohammad Mahdi Dehshibi, a postdoctoral researcher at the i_mBODY Laboratory in UC3M’s Computer Science Department, who led this study.
The research carried out by UC3M has developed a new method that uses a type of deep learning called s-RNNs (sparsely connected recurrent neural networks) together with GRUs (closed recurrent units), which are a type of neural network unit that is used to model sequential data. With this development, the team has managed to capture changes in pain-related body behaviour over time. Furthermore, it surpasses existing approaches to accurately classify pain levels and pain-related behaviour.
The innovation of the proposed method has been to take advantage of an advanced deep learning architecture and add additional features to address the complexities of sequential data modelling. The ultimate goal is to achieve more robust and accurate results related to sequential data analysis.
“One of the main research focuses in our lab is the integration of deep learning techniques to develop objective measures that improve our understanding of people’s body perceptions through the analysis of body sensor data, without relying exclusively on direct questions to individuals”, says Ana Tajadura Jiménez, a lecturer from UC3M’s Computer Science Department and lead researcher of the BODYinTRANSIT project, who leads the i_mBODY Laboratory.
The new method developed by the UC3M research team has been tested with the EmoPain database, which contains data on pain levels and behaviours related to these levels. “This study also highlights the need for a reference database dedicated to analysing the relationship between chronic pain and biometrics. This database could be used to develop applications in areas such as security or healthcare”, says Mohammad Mahdi.
These results of this research are used in the design of new medical therapies focused on the body and different clinical conditions. “In healthcare, the method can be used to improve the measurement and treatment of chronic pain in people with conditions such as fibromyalgia, arthritis and neuropathic pain. It can help control pain-related behaviours and tailor treatments to improve patient outcomes. In addition, it can be beneficial for monitoring pain responses during post-surgical recovery”, says Mohammad Mahdi.
In this regard, Ana Tajadura also highlights the relevance of this research for other medical processes: “In addition to chronic pain, altered movement patterns and negative body perceptions have been observed, such as in eating disorders, chronic cardiovascular disease or depression, among others. It is extremely interesting to carry out studies using the above method in these populations in order to better understand medical conditions and their impact on movement. These studies could provide valuable information for the development of more effective screening tools and treatments, and improve the quality of life of people affected by these conditions”.
In addition to health applications, the results of this project can be used for the design of sports, virtual reality, robotics or fashion and art applications, among others.
This research is carried out within the framework of the BODYinTRANSIT project, led by Ana Tajadura Jiménez and funded by the European Research Council (ERC) under the European Union's Horizon 2020 research and innovation programme (GA 101002711).